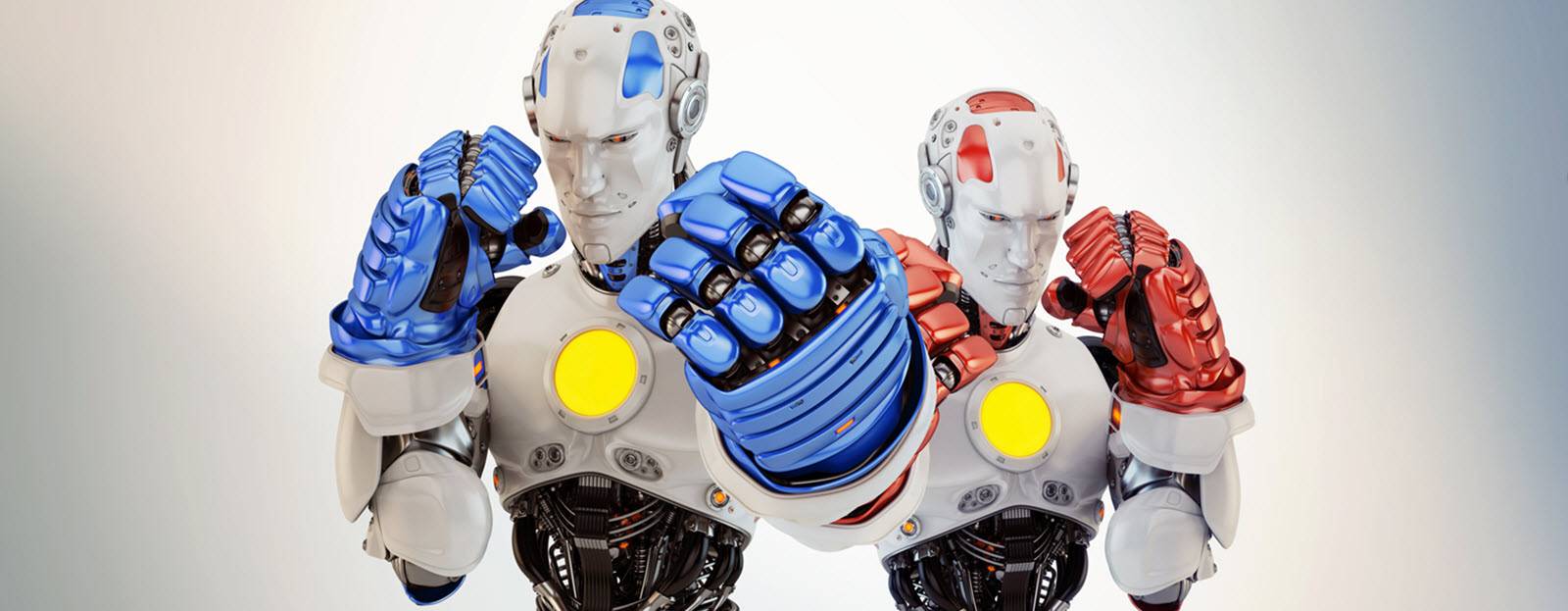
FSI (financial service industry) serves people and organizations, such as banks, money lenders, finance companies, real estate agents, and insurance organizations.
FSI dominates the world in terms of earnings and the value of equity market capitalization. Although there are some smaller businesses in this industry, most are dominated by large companies.
And yet, despite this apparent lean towards money-money-money, machine learning has already been put to good use—depending on one’s perspective—providing movie and advertising recommendations, as well as self-driving cars and trucks.
So, how can machine learning already be used to combat the pressing issue of climate change?
We can apply machine learning to improve Earth’s future climate projections by utilizing our abundance of historical climate data and observations—forecasts that will play a significant role in reducing our carbon footprint in the coming years.
Machine Learning: Our Best Bet To Grow
Machine learning is a subfield of AI (artificial intelligence). Although it has become somewhat of a buzzword, machine learning mainly refers to using data and algorithms to imitate the learning capabilities of a human.
To create a model, machine learning algorithms utilize datasets, either public or manually gathered, and can make predictions based on new data not included in the initial dataset.
Concerning climate change, there are two key methods that machine learning can use to better understand climate: observations and modelling. The amount of data available from observations and climate models has expanded dramatically in recent years. And, while human beings are unable to go through all the data in an effective and timely manner, machines can.
Forget The T-800
Unlike the terror imagined in the Terminator movies where the SkyNet AI becomes self-aware and tears down humanity with help from its T-800 cybernetic machine creations, the real-world machine learning aspect of AI can influence climate change—or more accurately, affect climate change decisions—that can save Earth.
There are three primary advantages that machine learning and its derivatives can provide to groups battling for the future of our planet:
More Accurate Predictions & Estimations:
Artificial intelligence and machine learning can be a suitable fit for data-rich climate science initiatives, such as those led by Google, the Institute of Mila (an Artificial Intelligence ecosystem, physically located in Quebec as a community of over 900 researchers specializing in machine learning), or the DLR (the Federal Republic of Germany’s research centre for aeronautics and space)—all of whom provide datasets of sufficient depth and diversity.
The resulting models created from aspects of their data can more correctly analyze consumption of energy levels in buildings and electric vehicles, making it easier to uncover trends and anticipate imminent usage. This eventually leads to efficient energy distribution and battery management, which are critical duties for those attempting to reduce their toxic waste and transition to renewables.
Among the deep data analyses in climate change research, is Facebook’s artificial intelligence-based population density map for most of southern Europe and deep convolutional neural networks used to detect extreme weather phenomena such as tropical cyclones, atmospheric rivers, and ice sheets.
Enhanced Tracking & Monitoring
Object detection and other computer vision technologies are utilized increasingly to boost the processing of visual climate data. Potential uses include tracking the extent of deforestation, producing size and position data for solar panels, and gathering data on weather adaptation policies across cities and states.
With climate change harming animals worldwide, conservationists require dependable techniques for tracking endangered species populations without disturbing them. Appsilon’s animal recognition project addresses this issue by developing an artificial intelligence model that can recognize specific types of animals in photos. The transfer learning technique is used to train a model, which provides higher accuracy even with minimal datasets.
Optimizing Legacy Systems & Workflows
AI solutions have demonstrated efficiency in reducing complex corporate procedures and improving older systems. In terms of “clean tech,” AI will be able to impact a variety of areas, such as environmentally-friendly buildings, sustainable supply chains, and freight transportation efficiency.
Innovations of this type are most prevalent in the agricultural sector. The advancement of artificial intelligence facilitates precision agriculture, which reduces waste and reduces carbon emissions while increasing agricultural production.
Machine Learning & Climate Change Symbiosis
While technologies to minimize climate change have been available for years, society has mostly failed to adopt them on a massive scale. While we expect that machine learning can help reduce the costs of climate action, humanity must also act.
There are numerous domains where machine learning could be used. These domains are classified according to the time frame of their possible influence and whether or not the technology involved is sufficient to gain particular benefits.
The main domains are: reliable electricity; low carbon materials; extreme weather condition predictions; increasing transportation efficiency; wasted energy reduction in buildings.
- Reliable electricity—electricity systems are “over flowing with data,” but not enough is being done to make use of it. By anticipating electricity supply and demand, machine learning could assist suppliers in better integrating renewable resources into national systems and reducing waste. The team at Google’s DeepMind UK lab has already used artificial intelligence to anticipate the energy output from wind farms.
- Low carbon materials—the manufacture of concrete and steel is estimated to account for eight to nine percent of total global greenhouse gas emissions. The development of low-carbon substitutes for these materials could be assisted by machine learning to reduce that percentage as AI helps scientists discover new materials by allowing them to model previously unknown chemical molecules and their interactions.
- Extreme weather condition predictions—it is believed that some of the most significant effects of climate change in the next few decades will be caused by extremely complex systems, including changes in cloud cover, and the dynamics of ice layers. These are the kinds of difficulties that AI excels at delving into. Modelling these changes will assist scientists in their ability to forecast catastrophic climate occurrences like droughts or hurricanes, which will provide global governments with the opportunity to better prepare for worst-case scenarios.
- Increasing transportation efficiency—some 25 percent of global energy-related carbon dioxide emissions are from the transportation industry, with road users accounting for two-thirds of those emissions. Machine learning, like electrical systems, can make this sector more efficient by decreasing wasteful journeys, enhancing automobile efficacy, and transferring some freight to low-CO2 choices like rail—however, electrification of the trucking industry may one day play a larger role than the early-stage self-driving vehicles.
- Wasted energy reduction in buildings—energy usage in buildings accounts for 25 percent of all worldwide energy-related carbon dioxide emissions and represents some of the low-hanging fruit for weather action. Part of the issue is that buildings are built to last, and even with renovations, aren’t always done so with high-tech features in mind. However, long-term gains can easily be reached with the simple addition of a few intelligent sensors to monitor air and water temperatures that could reduce energy usage by 21 percent in a building. Large-scale projects monitoring entire cities could offer a much more effective solution—anyone considering the development of a new city from the ground up?
Key Insights
As demonstrated, machine language can make significant contributions across a wide range of domains in the fight against climate change.
Using remote sensing, machine learning can enable automatic monitoring (for example, to locate deforestation, detect fire in an area, gather data on buildings, and assess damage after catastrophes).
Providing new materials for battery construction, carbon capture, and construction can help accelerate scientific discovery.
The machine learning process may improve efficiency, such as by consolidating freight, reducing food waste, and designing carbon markets.
In addition to enhancing physical simulations, hybrid modelling can reduce computational costs.
As a result, machine learning and artificial intelligence have shown great promise in transforming our lives, our work, the way we consume, and how it plays a role in how we grow our crops.